Unlocking the Power of ML for Business: A Beginner's Guide
Feb 23, 2023
5 min read
Machine learning is poised to transform the business world because of its capacity to process enormous volumes of data and find patterns and insights. This technology has the ability to spur growth, boost efficiency, and enhance decision-making in a variety of industries, from customer service to sales forecasting.

Businesses have already started using Machine Learning in their activities. The state-of-the-art model developed by OpenAI, ChatGPT, is one of the most well-known examples in recent times. Baidu, one of the largest technology companies in China, has a long history of investing in artificial intelligence and is reportedly launching a ChatGPT-style bot in March to merge with the company’s search engine eventually. In this blog, we will help you get started and unlock the full potential of Machine Learning Models for your business.
Basics of Machine Learning in Business
Machine learning is a subset of artificial intelligence that entails analyzing and understanding data using algorithms and statistical models and then using that understanding to make predictions or decisions without being explicitly programmed to do so.
To apply machine learning to business problems, you will need to follow these few (basic) steps:
1. Defining the problem: The first step is to clearly define the business problem you want to solve and how machine learning can be a part of it.
2. Gathering data: This is the most important step in integrating Machine Learning models in your business, collecting and preprocessing relevant data. This data will be used to train the model.
3. Choosing an appropriate algorithm: Then, you will need to select a suitable machine learning algorithm that aligns with the problem as well as the data.
4. Training the model: Train the machine learning model using the data. This process involves adjusting the model's parameters to minimize errors between the model's predictions and the actual data.
5. Evaluation of the model: Testing the model to evaluate its performance is an important criterion that will have a significant impact on your business.
6. Deploying the model: Implement the model in the business environment so that you can monitor its performance over time.
7. Refine the model: Fine-tune the model based on the results to improve its accuracy and performance.
It is critical to note that the success of a machine learning project in business is heavily dependent on the quality and quantity of data available, as well as the capability to comprehend and make decisions on the model's results.
You might say,“Oh, there are far too many steps involved in this; do you even know of any businesses that use Machine Learning in their enterprises?”
Real-world Examples of Machine Learning in Business and Their Impact
According to McKinsey, 49% of companies are investigating or planning to use ML, 51% of organizations claim to be early adopters of ML, 40% expect productivity gains from AI use, and 15% are already advanced ML users.

Here are some real-world examples of how businesses are benefiting from incorporating Machine Learning:
1. Amazon's recommendation engine: Amazon uses machine learning to provide personalized recommendations to customers based on their past purchases and browsing history. This has increased customer engagement and sales, making Amazon one of the largest e-commerce companies in the world.
2. Netflix's content recommendation: Netflix uses machine learning to recommend TV shows and movies to its subscribers based on their viewing history. This has improved customer retention and engagement, helping Netflix to become the world's largest streaming platform.
3. Google's search engine: Google uses machine learning to provide relevant search results to users based on their queries. This has improved the user experience and increased the efficiency of the search process.
4. Shazam’s music recognition: Shazam uses machine learning algorithms to quickly and accurately recognize the music playing in the background of a user's environment. By leveraging the power of AI, Shazam has been able to improve the user experience, increase engagement, and drive growth. Personalized recommendations have helped Shazam become a trusted source for music discovery and recommendations.
5. Salesforce: Using the vast data sets that Salesforce's CRM software collects on potential customers, machine learning can identify patterns of customer behaviour that are too complex for a human to detect. Customers will increasingly look to Salesforce to help them manage their sales process and help them decide which customers to invest in and which to de-prioritize. As a result, SalesForce is the world's leading CRM platform.
Fraud detection, predictive maintenance of equipment, and healthcare are just a few of the industries that are starting to see the benefits of utilizing Machine Learning in their operations.
“Okay, so what you’re saying is that I need to integrate Machine learning models in my business in order to stay ahead and outperform other businesses ?” Yes and no.
To use or not to use ML?
While machine learning has countless advantages for businesses, it is not always the best solution for every problem.
1. Data Availability: Large amounts of data are required to train models and make accurate predictions in machine learning. If a company lacks access to relevant data, it may not be a good candidate for machine learning.
2. Problem Complexity: Complex problems that are difficult to solve using traditional methods can be handled by machine learning. If a company faces a complex problem, such as predicting customer behavior, machine learning may be a good fit. If a problem is simple and can be solved using traditional methods, machine learning may not be necessary.
3. Return on Investment (ROI): Machine learning can save money and increase efficiency, but it requires an investment in hardware, software, and personnel. If a company cannot afford the investment or the ROI is unclear, it may be better to wait rather than jump on the bandwagon.
4. Time Constraints: Machine learning models can take time to develop and deploy. If a business needs a solution quickly, it may be better to look for alternative solutions.

In general, if a company has a complex problem to solve, access to relevant data, and the resources to invest in hardware, software, and personnel, then it should invest in machine learning. On the other hand, one should not invest in machine learning if it lacks high-quality data, has a simple problem that can be solved using traditional methods, or cannot afford the investment.
Future of Machine Learning in Business and How to Stay Ahead of the Curve
Some strategies for staying ahead of the curve include:
1. Integration with other technologies: Machine learning will increasingly be combined with other technologies, such as cloud computing, the internet of things, and blockchain, resulting in new and more sophisticated solutions.

For instance, Predictive maintenance is a technique that uses machine learning algorithms to predict when a piece of equipment is likely to fail, allowing maintenance to be scheduled in advance. This reduces downtime and increases operational efficiency. This technique is used by many industries, including manufacturing, healthcare, and transportation, to improve operational efficiency and gain a competitive advantage.
2. Focus on explainability and trust: As machine learning algorithms become more prevalent in business decision-making, there is a growing concern about the potential for these algorithms to make biased or unfair decisions. This has led to increased scrutiny from regulators, customers, and other stakeholders, who are demanding greater transparency and accountability from businesses that use these algorithms. For example, in 2018, Amazon developed a machine-learning algorithm to help with the recruitment process. The algorithm was trained on resumes submitted to the company over a 10-year period. It was designed to predict which candidates were most likely to be a good fit for Amazon's open positions. However, the algorithm was found to be biased against women, as it was trained on a predominantly male dataset and therefore favored male candidates. This resulted in a higher rejection rate for female candidates and a lower representation of women in the recruitment process. Amazon had to rethink its approach to the use of machine learning in the recruitment process. The company implemented measures to ensure that the algorithms it used were transparent, explainable, and fair, and it worked to build a more diverse dataset that reflected the diversity of the candidates it was seeking to hire.
3. Personalized experiences: Machine learning will be used to create highly personalized experiences for customers, such as personalized recommendations and marketing campaigns. Meta is now leveraging data from users' search and browsing history to understand their interests and preferences in order to provide personalized recommendations across their platforms. This information is then used to train machine learning models that can recommend content, products, or services that are likely to be of interest to the user. The impact of this machine learning-powered recommendation system has been significant for Meta. By using machine learning, Meta has been able to improve the user experience, which in turn led to increased revenue and growth for the company.
Conclusion
To summarise, machine learning has the potential to revolutionize the way businesses operate and solve problems. Businesses can use machine learning algorithms to optimize processes, make more informed decisions, and provide personalized experiences to customers by leveraging the power of data. Businesses must focus on identifying the right problems to solve, building a strong data foundation, and prioritizing explainability and trust to get the most out of machine learning. Machine learning, when used correctly, can help businesses stay ahead of the curve and achieve their goals in the coming years. Companies that embrace machine learning and invest in technology will be well-positioned for success in the years to come.
Written By
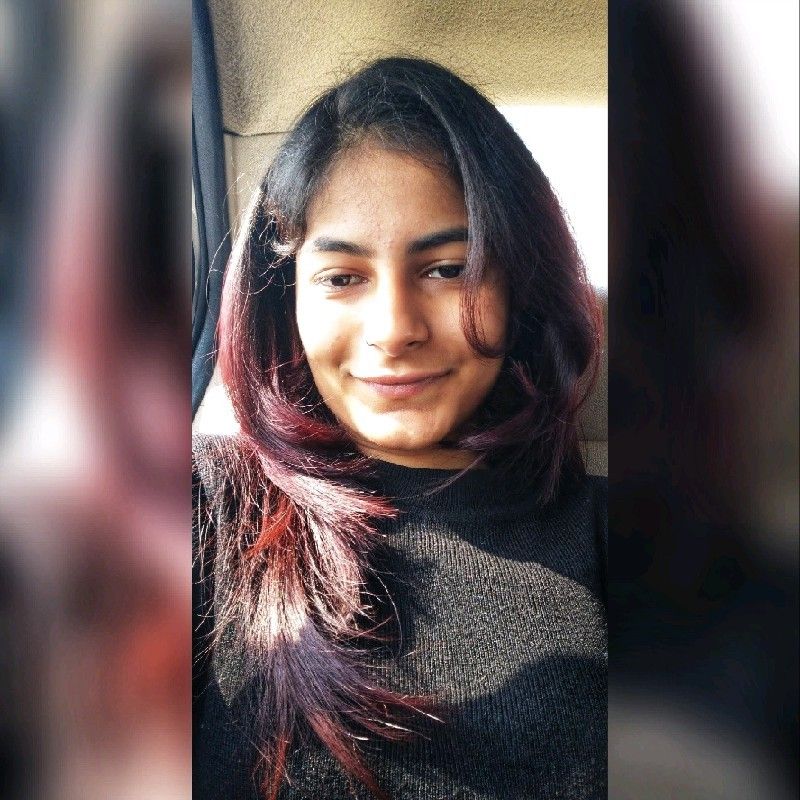
Wamika Jha
Technical Content Writer