Maximizing ROI with MLOps: How NimbleBox.ai Can Help Your ML Team Drive Business Outcomes
Apr 14, 2023
5 min read
Are you a machine learning startup or just a tech startup that is expanding into ML? What is the first step in your new endeavor? Well, it is ideation, but what after that? Deploying or, instead, the entire MLOps cycle can cost your team thousands of dollars in hardware, software, salaries, and whatnot! So how do you judge if that cost is valid for what you will receive?
Return on Investment is the primary performance or efficiency measure expressed as a ratio or percentage which helps a company or a team determine if a new endeavor will be helpful in business. Like any other business, predicting or assuming returns before even starting the production process is essential, as it gives value to the time and money spent. So let us explore how you can maximize your ROI with NimbleBox and drive your team in a pro-business direction!
The Challenges of Achieving ROI in Machine Learning
With all of the world suddenly using Machine Learning in their businesses, you may be wondering about adding it to your pipeline, but what you or the thousands like you aren’t thinking about are the unseen barriers and challenges that come with introducing a never-ending cycle of data acquisition, model training, and monitoring that goes into deploying such a system.
Let us look at some of these challenges. Maybe it will help you find the answer as to why your ROI lags in comparison to others:
1. Old Legacy Hardware: Most of the time, enterprises may choose to shift efforts toward ML attracted by the promise of automation and efficiency it offers but may need more the very fundamental infrastructure requirements of such models.
Legacy Enterprises, however, come with a vast plethora of historical data. Still, when it comes to being technically sound enough to make that profitable for the company by properly engineering it and then training them on last-generation computers, all the advantage that came with the data just goes down the drain.
Even if you are training your model on the cloud, multiple steps in a pipeline require sound systems that can perform tasks like creating scripts and various API calls.
2. Insufficient Data: Now you have the newest systems loaded with the beautiful 12th Gen Processors and 3080s; what is the next big issue you will run into trying to fish ROI from your pipeline? Acquiring data is one of the most tedious jobs when training these new-age Large Deep Learning models.
Not only the various legal guidelines pertaining to collecting data, but we also need a friendly and efficient infrastructure to collect vivid data arrays that are usable and workable for your Data Scientists and Analysts. If you wanna learn more about data management, how about going through “Best Practices for Managing Data in an MLOps Environment” by Nimblebox to gauge and course-correct your data management skills?
3. Isolation from End User: Machine Learning won’t turn into MLOps until team communication channels are set. What we mean by that is if your machine learning team is totally isolated from what your enterprise is trying to achieve, for example, a team of DL experts working on a Geo-Spatial Imaging Project led by Environmentalists, without the actual industry experts helping the team or lack of such individuals will affect the ROI for your ML Endeavor.
Such isolation from industry experts is bound to cause a disconnect between what the ML team understands is ideal for the model’s performance and what is required to make the entire operation profitable.
4. Lack of ML Professionals: An isolated ML team will still produce results given the adequate data (not the best, perhaps), but you know what won’t? A lousy team of ML Professionals is the leading root cause for your ROI not reaching the mark you want it to.
A talented team empowered with knowledge and experience can help you bypass all the above three challenges with innovative solutions. For example, a technical talent that knows how to deal with hardware challenges, cleaning and making datasets, and someone who Would see the problem statement as a purely mathematical problem and make the model the best version of itself is something that will immediately shine in your ROI.
Check out this guide to “Setting up your MLOps Team” to know what talent to scout for when trying to make ML profitable!
Now let us see how having an MLOps platform can help you address these challenges that limit your ROI.
How NimbleBox.ai Can Help Maximize ROI
NimbleBox.ai, or any MLOps platform, can make your pipeline shine and help maximize your ROI. MLOps platforms have various plugins and services to help automate smaller and more complex aspects of your machine learning pipeline. Such a platform can also allow you bypass the challenges of Legacy Systems and Data Management.

Let us look at some parts where such platforms shine and why they should be integrated into your pipeline:
1. Deployment: Having a well-integrated platform helping you manage your Kubernetes Clusters can help you deploy models at an incredible pace, promoting a new level of scalability, which significantly cuts time consumption into figuring out load balancing. Such systems can handle deployment tasks like this, helping your team focus on Monitoring and Development.
2. Monitoring: Such a platform helps monitor models with good algorithms and well-tested practices like A/B testing and the other evaluation metrics that can be implemented to understand how your model is performing in the wild. Such monitoring can help you course-correct your model at aspects where it needs to be, increasing your ROI.
3. Communication: With various provisions to control access and share resources and files over a supervised platform, the communication among teams becomes seamless and minimizes the isolation among team members, maximizing the efficiency of development. Such communication helps increase ROI by bridging the gap that often causes delays in deadlines.
4. Value to Business: Quickly becoming the first asset that needs to be a part of a Machine Learning enterprise, the MLOps platform adds value to the entire machine learning pipeline by addressing issues like collaborating, testing, releases, monitoring, etc., through reliable and tested techniques.
We have seen the broader things having an MLOps platform helps with in terms of increasing ROI for your company, let us now see individual elements of NimbleBox.ai that will help you maximize your ROI to the absolute best!
Best Practices for Maximizing ROI in ML with NimbleBox.ai's MLOps Platform
Now that we have listed the different causes holding you back from maximizing your ROI, and how having an MLOps platform can help speed things along. Let me take you through the various ways where we, as NimbleBox, can help you maximize your Return on Investment.
NimbleBox, over the years, has been developed by ML Engineers figuring out solutions and tools and further perfecting these tools that intrigued us to come up with different modules that can assist different aspects of your machine learning enterprise. Let us look at some of these modules and how you can utilize them to maximize your ROI.
Build: It is a plug-and-play playground for data scientists to build and train their models without worrying about the underlying architecture, making your experimentation fast and easy. Having been used by over 15,000 developers across leading firms worldwide, Build by NimbleBox.ai is our most battle-tested product.

Scaling your team also means inculcating accountability. This feature empowers accountability in your team by providing insights into your hardware and storage usage, viewing project runtime, and checking your team members' activity in the workspace. For this particular reason, you will be able to tackle the Communication and Monitoring challenges that may be bringing your ROI down.
Deploy: Automate your deployment process to be a safe and smooth process by using Deploy by Nimblebox. With nifty features like deployment id and workspace id, you will be able to track the deployment progress and stage.

The authentication process makes it so only the right people who have gone through all the reviews and testing are able to deploy the model. This module will help you optimize your Deployment to the fullest.
Jobs: Jobs help automate redundant tasks and schedule the job at a particular interval. Such jobs are able to save you a bunch of money by automatically closing the instance when you want it to.

With jobs having total control over the instances, be it alive or dead, you will be able to backtrack to any training instance in steps that are even lesser than backtracking a Git file. This module really helps you tackle Monitoring and Adds Value to your Model Training.
Artifacts: Relics is a new object storage integration feature introduced in NimbleBox.ai, specifically designed to handle large amounts of data utilized for your machine learning workflows.

Relics provides the ability to store various forms of unstructured data (any type of files) in the NimbleBox object store or integrate with your cloud object stores, such as Amazon S3, GCP GCS, and ABS Azure. This means you can keep your data safe and easily accessible, without having to worry about losing it or running out of storage space.
These modules are continuously under rigorous testing and development, and are made for Machine Learning Engineers by Machine Learning Engineers.
Conclusion
As a startup looking to expand into machine learning, investing in the entire MLOps cycle can be daunting, with significant financial and resource implications. However, by leveraging the power of Return on Investment, you can make a data-driven decision that maximizes the value of your investment.
With NimbleBox, you can access cost-effective solutions that simplify the process of deploying machine learning models, allowing you to focus on creativity and other critical aspects of your business. By partnering with NimbleBox, you can take the first step towards creating a successful machine learning startup and driving your team in a pro-business direction.
Written By
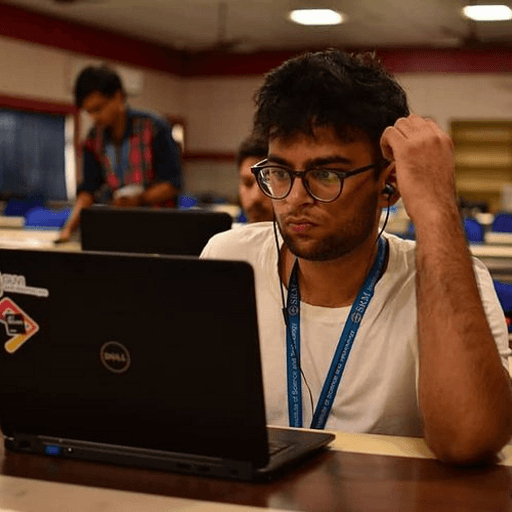
Aryan Kargwal
Data Evangelist