In the rapidly evolving world of Machine Learning and Artificial Intelligence, the advent of Large Language Models (LLMs) has been nothing short of a revolution. Among the pioneers in this field, META's open-sourced LLM, known as LLaMA, has been a game-changer, purely because it made such models not just a myth or mystery to the average Joe but something that can be fine-tuned and deployed according to their needs. Today, we stand on the brink of another significant leap forward by introducing LLaMA 2, the successor to the original model, now supercharged with an even more significant number of parameters.

In this Blog, let us look at the enhanced abilities of LLaMA2, how it bodes against its predecessors and competitors, how you can access LLaMA2, and where all this is headed with this model being Open-Sourced.
What is LLaMA 2?
Following the Open-Approach Meta implemented for LLaMA, their take on Foundational Large Language Models, which came as an answer to the strictly moderated and secretive ChatGPT and GPT-4 by OpenAI, Meta AI has just released the second iteration to the industry-shaking architecture.
LLaMA 2 is Meta AI’s freely available model for research and commercial use (one of the key factors making it different from OpenAI’s GPT Series). Ahead of the success LLaMA got due to the sheer resources and channels available to fine-tune the model to custom use cases, over 100,000 requests to access the weights, and countless new variants in the market, Meta has decided to capitalize on the revolution by teaming up with Microsoft, as announced at their latest Microsoft Inspire making Microsoft the preferred partner for LLaMA 2.
How good is LLaMA 2?
With the initial strategy of having different variants of LLaMA from the get-go, them being LLaMA 7B, LLaMA 33B, and LLaMA 65B, Meta AI has always been putting a preface on smaller models trained on more tokens, which has been a defining feature making even locally deployed models to have performance which will be somewhat comparable to the ones generated by OpenAI APIs.

Now where does this already strong foundation with LLaMA bring about for LLaMA 2? LLaMA 2 has received over 40% more data than its predecessor to train upon and can process double the context. The model comes in 3 public and private releases, LLaMA 2 7B, LLaMA 2 13B, and LLaMA 2 70B being the public ones, and LLaMA 2 34B being the still closed source one. LLaMA 2 has trained more than 1 million human annotations.

When put against the existing big players, be it the GPT models or the models that have been fine-tuned upon the existing open-source LLaMA model, we can see a clear and significant drop in the safety violations by the variants, which can be thought of as the perfect metric to check LLMs given they are intended to generate text like a human would. Taking a sample size of ~2000 adversarial samples generated in both uni-prompt and multi-prompt scenarios, we can see how the variants are performing even better than ChatGPT, Vicuna, PaLM Bison, MPT 7B, etc.
Open Source vs. Closed Source LLMs
Now, the performance aside, LLaMA rose to popularity due to Meta AI’s decision to make the model and its variants Open-Sourced. This, in turn, gave us many impressive architectures and models, all fine-tuned and built upon LLaMA, which (high chances are) your favorite LLM Platform must be using. Let us see the difference between having your LLM Open Source vs. them being Closed Source.
Open Source LLMs:
From an ethical standpoint, open-source LLMs promote transparency and collaboration. They allow anyone to inspect the code, which can lead to identifying and rectifying biases, errors, or security vulnerabilities. This transparency can also ensure that the technology is being used responsibly.
From a practical perspective, as a user, you can modify and adapt open-source LLMs to suit your specific needs. However, this comes with the caveat that you need the technical expertise to do so. Additionally, while the model itself might be free, running it (especially at scale) can require significant computational resources, which can be expensive.
Closed Source LLMs:
Ethically, closed-source LLMs can be a mixed bag. On the one hand, the organization that owns the model has complete control over its use, which can help prevent misuse. On the other hand, the lack of transparency can make identifying biases, errors, or security vulnerabilities challenging.
As a user, closed-source LLMs are easier to use, mainly if the owning organization provides an API. You won't need to worry about the computational resources required to run the model, as the organization typically handles this.
Accessing LLaMA 2
LLaMA 2 has been made publicly available by Meta on their [website](https://ai.meta.com/llama/) for research and commercial use, which can be found on this link. Now, however, if you are just looking to check the model out and playtest the model’s limits, how about going to chat.nbox and checking out LLaMA 2 13B right now?
Future of Large Language Models
According to us, the Future of LLMs should look like a complete Democratization in the field of AI. The democratization of AI refers to making artificial intelligence technology more accessible and available to a broader range of people and organizations. Historically, AI development was primarily restricted to large tech companies, research institutions, and well-funded organizations due to the significant resources, expertise, and infrastructure required.

However, the democratization of AI seeks to break down these barriers and empower a more diverse set of users to leverage AI capabilities for various applications. Here's how this democratization process unfolds:
1. Ease of Use: Democratization involves creating user-friendly AI tools and platforms that do not require advanced programming skills or specialized AI knowledge. This means developing intuitive interfaces and tools that allow non-experts to interact with AI technology effectively.
2. Pre-built Models and APIs: Offering pre-trained AI models and APIs (Application Programming Interfaces) allows developers to integrate AI capabilities into their applications without building models from scratch. Cloud-based services like Google Cloud AI, Microsoft Azure AI, and Amazon AI are examples of platforms that provide such services.
3. Open-source Frameworks: Making AI frameworks and libraries open-source allows developers and researchers to access, modify, and contribute to developing AI tools and algorithms freely. Examples of popular open-source AI frameworks include TensorFlow and PyTorch.
4. Affordable Infrastructure: Cloud computing has played a significant role in democratizing AI by offering affordable computational resources. Users can rent computing power on the cloud without investing in expensive hardware, making AI experimentation and deployment more accessible.
5. Small and Medium-sized Enterprises (SMEs): Democratization enables smaller businesses and startups to leverage AI technology to improve their products and services, fostering innovation and competition.
Overall, the democratization of AI aims to remove barriers to entry and empower individuals, businesses, and communities to use AI technology creatively and responsibly. By making AI more accessible, it has the potential to drive innovation, improve efficiency, and address real-world problems across various domains. However, it is also responsible for addressing potential ethical concerns and ensuring that AI is developed and deployed responsibly.
Conclusion
In conclusion, Llama 2 represents an exciting leap forward in the world of large language models, marking a significant milestone in the ongoing democratization of AI. Meta AI's dedication to building upon its earlier open-source model, Llama, showcases a commitment to innovation and accessibility within the AI community.
With Llama 2's enhanced capabilities and increased scalability, we can expect more robust and contextually aware language models to tackle complex natural language processing tasks with remarkable accuracy. As AI technology continues to evolve, the potential applications of Llama 2 are limitless, from powering interactive chatbots and personalized virtual assistants to revolutionizing content generation and text analysis across various industries.
Written By
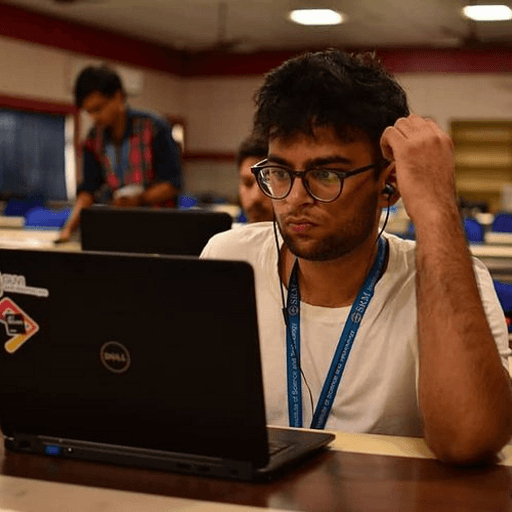
Aryan Kargwal
Data Evangelist